.png)
The whole world seems to have been bitten by the generative AI bug. It has definitely generated a lot of headlines, from ChatGPT’s meteoric ascent to software companies of all stripes adding features driven by generative AI.
It is natural to assume that generative AI will emerge in accounting software – but in what form?
Intuit was very early off the mark with Intuit Assist, a personal assistant spanning multiple Intuit programs that can carry out a number of tasks. But Intuit Assist could do much more interesting things in the marketing platform MailChimp than it could in QuickBooks.
I had a long, open discussion with Kendra Vant, who was the executive general manager for data and AI products at Xero until August this year. Vant worked across Xero’s commercial data strategy, AI product portfolio and investment roadmap, AI literacy, and responsible data use by both Xero employees and customers.
In her role at Xero and previously recruitment ads platform Seek, Vant released multiple AI products to market and generated key patents. She has a PhD in Physics from MIT and is an adviser to AirTree Ventures, one of the most prominent VCs in the Australian market, and now consults under Europa Labs.
Vant is also known for her stance on ethics in AI. She established and chairs the Responsible Data Use Advisory Council, comprised of experts and thought-leaders globally, which aims to set best practices in data sharing and leveraging data to benefit small businesses and their advisors.
We had an open discussion about the potential, the limitations and the commercial aspects of generative AI, and how it may influence the direction of accounting software.
This interview was edited for clarity and length.
DigitalFirst: I want to ask you about generative AI and it’s impact on accounting software. There’s a lot of hype right now.
Kendra Vant: I think everyone is so incredibly excited about this AI technology because it's talking to us. Six months ago when it was really hitting the mainstream, I had lots of excited and interested friends and acquaintances who would usually be really hard nosed commercial people saying things that made no sense at all. Like, dudes, you know, there's no free lunch. You know this is going to cost an arm and a leg.
And I tried to figure out in my own head, why people were so willing to just sort of almost be childlike in a way that they're not usually with new technology.
And I think it's because it's natural language. And we find it very difficult as humans not to anthropomorphise something because it speaks to us in really good, literate English. So that's kind of curious. It does make me think that there will definitely be really great use cases as an interface language, as an interface layer.
DigitalFirst: So let's start with that. I assume you've seen the videos of Intuit Assist.
Vant: I've seen it to the level that I've seen Microsoft's agent and Google's agent. And everybody's coming out with very beta, very early versions of a personal assistant. None of them are very easy to fiddle with. And they're all looking interesting.
Intuit released a preview of its personal assistant, Intuit Assist, which works in QuickBooks, Mailchimp and TurboTax
DigitalFirst: Do you feel like that is an inevitable endpoint for interfaces with business software and accounting software? Will we all end up using chat interfaces, either typed or spoken?
Vant: Yeah, I don't know. I don't think anybody does. Because I don't think anyone knows if that's how we actually want to interact with them.
I think that large language models have certainly given us an opportunity to have another crack at the idea that everybody wants to use chatbots. Because the previous versions were pretty bloody clunky. And this has the opportunity to make them not clunky.
Do we want to do those things through chatbots? I'm not sure. Because sometimes we want to have conversations with things and sometimes we want a structured way of interacting – with something like a form where we just get stuff done.
I'm not a bookkeeper and I'm not an accountant, but it's not the most obvious one for me where I definitely want to be chatting.
And is it feasible? I don't know. We're still quite a long way off solving hallucination problems. And some of the ways that we're solving those things, people have been commenting on the fact that (Chat) GPT4 feels like talking to a Boy Scout. Because they are having to clamp down so heavily on the creative part of a large language model to stop it saying things that are either incorrect, offensive, or just made up.
There’s a lot of people in the more techie side of the world are saying, I want to talk to (ChatGPT)3.5 because it's kind of an idiot but at least it sounds like it's got a soul, right? Whereas (ChatGPT)4 sounds very straight-laced.
Even just an interaction with the algorithms now, we're starting to see that as you squeeze in one place, they pop out in another place.
DigitalFirst: So even if you could have the perfect chatbot personal assistant, you may not want your business software, using that style of interface? I'd imagine, for example, Excel will be difficult. Sometimes you would just want to drag and drop stuff around as opposed to trying to give instructions vocally or typing in directions.
Vant: I think so. When I watch sci-fi movies and they all throwing this here, that and the other, I'm like, well it will work for some things, but for other things it'd just be bloody annoying. Because sometimes I want to be really precise.
So will they get good enough to the point that you can actually be really precise? Maybe, but I don't think it's going to happen super quickly.
And for folk like me anyway, I have an accent that's not the standard accent. So you said chatbot or voice bot. We saw Spotify add translations of podcasts into other languages. And that was cool. But it’s stitching together technology that has existed for a while. Speech to text isn't new. And yet I don't find the speech to text interfaces with the Google and Amazon devices in my home very reliable because I don't enunciate very well and I'm not an American. So It's really irritating.
Will they get better? Yes. My gut feeling personally is generative AI, which at the moment costs a hell of a lot – environmentally and from a financial point of view – will be incredibly ubiquitous in 15 years. It will do more than we think it will. But it will take longer than we think it will.
You're already seeing Google saying, don't expect us to make a lot more money, and Microsoft saying, We're not going to make more money out of this in the short term, because it's so bloody expensive.
I am worried about the trough of disillusionment as everybody who doesn't understand how technology transformations work expects massive change in the next six months, and then get pissy about it and say, “But you promised us a rainbow”.
DigitalFirst: Do you think it's too early for companies like Intuit to come out and start making bold promises? Sasan Goodarzi, Intuit’s CEO, did this big announcement around Intuit Assist, I think it's still in closed beta, but they’re making bold promises.
Vant: I don't think we penalise CEOs for making those kinds of announcements. Okay. We see CEOs do that all the time. And I think that they do it because we don't penalise them. We're perfectly happy for them to say things like that.
DigitalFirst: And so interface is one thing. Being able to control the program, find settings, change things. What about asking generative AI to create things? Like reports, I guess.
Vant: So you're talking about the differences between something that assists you to operate the software as it already exists, versus the more creative, summarisation stuff?
DigitalFirst: Yes.
Vant: Yes, there’s using generative AI as the chatbot layer to interface with the human so that the human can be walking the dog in the park and just talking to the phone.
That is certainly feasible. There will be the inevitable challenges of natural language being natural and it isn't completely structured. Anything that saves me time sounds good.
I am not an accountant so I would leave it to them to suggest with how useful it will be to have something that can go in, read lots of textual information, summarise that into a good first draft.
My gut feeling is that it will be super helpful, particularly if there is writing that is relatively generic. If you do it a lot, that's boring. And humans don't like boring.
As a non-accountant, the idea that you'd be able to go and look at different, important, personalised sources of information about a particular client and ask the assistant, can you synthesise a good first draft? That, in my opinion, is fully within the capabilities of what's in market at the moment in terms of large language models. And everybody's going after that, right? There are 1,000 startups doing some form of first draft.
Do I think that first drafts will be better from companies that are focusing on a specific problem subset like say, for instance, creating summaries of your inventory, or your sales or something like that? Yes, I do. There's definitely space to play for those companies to go hard for making this work really well, to really tweak it and really steer it and make it sound like an accountant sounds.
I don't think it's going to be won by a single summarisation engine that creates all kinds of writing. Now you'l be damn sure Google and Microsoft will both be trying. And they're already launching those things, right? “We can be your assistant and do everything.” We're also seeing how much those things cost. They're losing money on those products.
So people are saying, do you really need to have the all-singing, all-dancing LLM, which costs a lot every time you call it to solve every problem? Or will it be more cost effective to make more specialised, smaller and cheaper LLMs to do things like that? So I think there's a hell of a lot of people trying out those ideas at the moment,
DigitalFirst: Maybe there'll be a broker LLM that will classify your problem and then send it off to the cheapest LLM you can use to solve their problem?
Vant: There are some people already certainly talking about that. You know there's this thing in machine learning called a mixture of experts. You effectively have specialised models behind the scenes and a master model that decides which one to use.

DigitalFirst: Accounting software has used machine learning (a different type of AI) for a long time to match bank transactions. Can you use LLMs as a kind of fuzzy logic to improve matching?
Vant: Matching? LLMs don't work like that at all.
DigitalFirst: Well, an LLM predicts the next best word or next best letter. And they’re drawing from a pool of data of examples to work that out. Bank rec matching in Xero is amazing compared to where it was, but it’s not perfect. Is there an opportunity to use LLMs, generative AI to improve that somehow?
Vant: So generative models in general, of which large language models are the language ones, are doing exactly what you said they're doing. At the moment, people are wrapping all sorts of stuff around them. The plain ones are generating the next token (word or character) based on the statistical likelihood of that sequence appearing.
But I think it makes you think that they're going out all the time, scanning across all of their databases and saying, “I see the sequence five times, so I'm going to put that”. And they don't (work like that).
When you train the models, they learn the likelihood of that thing to happen. And then they're just running numbers. They're doing massive matrix multiplications to say, which one of my weights fires next, which pathway do I go down on the generation path. So they're absolutely not matching.
It's an interesting question (about applying generative AI to bank rec). So you hit upper limits and accuracy in things like matching tasks. Often because of stuff in the data, or because different humans want to put them in different places.
I might decide that this goes into this general ledger code, you might decide that it goes into a different general ledger code – and we're both right. And that's when you start to hit the limits of how good you can make machine versions to replace the human when the humans stop agreeing. Or when I spell (hardware retailer) Bunnings one way and you spell Bunnings a different way. And when you know the optical character recognition on the invoice screws up and doesn't give us the information we need. So that's why those things hit fundamental limits. And why you say they're good but they're not perfect, which I agree with.
And there's also the challenge in small markets, if you just enter a market you don't have much data in that market yet. You don't know the vendors in that market. So there's always edge problems and teething challenges and all those sorts of things.
DigitalFirst: So if I reframe it – Xero and Intuit are sitting on massive piles of bank transaction data, they've been applying these machine learning algorithms to get the best matches on bank rec. If I were to apply LLM to that big pool of data, would they get a better result or is this the wrong kind of application?
Vant: Is somebody trying it? I'm sure they are because you might as well. It's actually quite hard to say that something won't work.
I don't know, is the answer. Would you try it? You'd probably try it. Is it obvious to me that it's going to clean up the edge cases? It's not obvious to me that it will, no.
DigitalFirst: So there is so much potential and it is still so uncertain as to what the outcome is going to be. I'm just wondering, do you think that having some experience, having the ability and the budget to explore with an LLM is going to be essential for the next generation of business software? Can a vendor just afford to ignore it?
Vant: If you're building software that you expect to be used by thousands or millions of people I'd certainly be looking at it. And it's not that expensive now. Because there's a massive, massive difference between training it and using it. Most software vendors are not going to go and train their own. That's why they're called foundational models. And the people have been quite clever about choosing that terminology.
I personally think things happen slower than we think they will. And you don't see the enterprises adopting this stuff yet. You do see it coming in already in terms of prewriting and summarisation into a lot of SaaS software.
I think in five years, most people who try to build consumer software will have large language models somewhere in their space. If I was in such a company, would I be making sure that some part of my workforce was becoming really literate in that space? Absolutely. Because I don't think that cost of running the LLM is the bottleneck. I think it's going to be people who understand how to put them to use.
DigitalFirst: So this is a great point to clarify. Karbon are using generative AI for drafting emails and summarising email threads. Intuit seems to have more control over its own. I don't know if Intuit has built a foundational AI.
Vant: Would they have trained their own? To the very best of my knowledge, the only companies that have trained their own foundational models are the cloud companies. Meta has, Google has, Open AI – which is effectively Microsoft – has. Anthropic has just formed big deals with Amazon. There's a French startup that's trained its own. And it costs tens of millions, hundreds of millions of dollars to train them. Everyone else to the best of my knowledge is sitting on someone else's model.
DigitalFirst: The Intuit CEO said that they had trained on their data…
Vant: But that’s a different thing, Sholto. Companies are picking up foundational models which have been trained on literally web-scale data. What that training has done is basically taught syntax and grammar and sentence structure. It's taught it to have a conversation. Then people are picking up those models and they are doing one of two things. They are fine tuning those models with their own corpuses of data. Or they're doing what is called prompt engineering.
And they are really heavily focusing those models in one of those two ways to make it more suitable to a really specific task. So I'm 99 percent sure that what you heard the Intuit CEO say was that they have fine tuned and trained models with Intuit customers’ data to become more appropriate for accounting services, but I very much doubt that they trained their own. I thought they had a partnership with open AI. (ED: Vant was correct – Intuit announced a partnership with Open AI in June this year.)
DigitalFirst: So foundational AI is going to be a cost issue. But if you're using a foundational model from someone else, then there is actually the potential for anyone really to start using it.
Vant: There's two different ways in which these things are expensive, right? There's training them and there's using them. And until up until the the advent of really large language models, the cost of training things were quite expensive. And the cost of using them, which is called inference, is usually cents on the dollar, it's pretty cheap. It's not free. But it's not “We have to charge a lot for that product to make it worthwhile”.
The biggest, coolest foundation models – so GPT4, and Google’s Gemini, which Google said they're going to release in a few months – the cost of running those is material. So the inference cost of running those models is actually really significant. Which is why Microsoft and Google have said, they're losing money on copilot and things like that.
So it's not going to be prohibitively expensive because I can take someone else's foundation model and I can fine tune it myself. It's still going to cost maybe hundreds of thousands or millions of dollars – difficult for a startup to do. That's not fair, you can do it for cheaper than that. So that's not going to be cost prohibitive.
When your customers are going to throw heaps of (tasks) at you, that might become cost prohibitive, you might charge quite a lot for your service to make that a financially viable product.
DigitalFirst: So if Gen AI becomes an intrinsic part of accounting software, you'd expect the vendors to have to increase the cost of it at some point to cover it?
Vant: It's a really interesting question. Are lots of really clever, dedicated people working to bring those costs down? Absolutely. But it's not a free lunch. So when I hear people talking about saying, Hey, we're going to replace all our customer service agents with chatbots, I ask, have you checked to see whether that's cost effective? Because you don't pay customer service agents terribly much. It's not 100% completely obvious that it's going to be cheaper to replace those with the people you need to build the software, that you need to maintain the software and then run all the software. It probably is. But will they will they have to raise their prices? Because the stuff they run is really expensive? I guess it depends how deep your pockets are. I mean, Amazon and Google are prepared to run at a loss, clearly. Itt could certainly change the cost of delivering the service.
DigitalFirst: I guess there's a cost of training if your data set is extremely large as it is for Intuit and Xero. Will there ultimately be a question as to who has the deepest pockets to do this successfully? Who's got the biggest R&D budget? It’s one thing to summarise email threads and generate emails, but that's different to asking it to bring in large numbers of transactions and create a particular type of report. I would imagine that that would use a lot more tokens.
Vant: You can sell it as a premium service. Because it's not R&D. That's that's the cost of running the service.
DigitalFirst: You're right – that is the cost of running the service, But the whole fine tuning process, I just imagine that it rises with the volume of data.
Vant: It does rise with the volume of data you have. Absolutely. And so you'll spend lots of time being clever and savvy about how much of the data you actually have to use. But again, the constraints are more likely to be who can attract the people who can build the stuff rather than just how much money have I got in compute. Now if you're trading a foundational model, then you need so much money and compute that's a whole company in and of itself. But if you're doing a fine tuned model, my gut tells me that the people who would build the stuff would still be more expensive than the compute you needed to train it.
DigitalFirst: Okay, cool. Any final thoughts on the future of accounting software? I mean, it is so early, but it is worthwhile trying to imagine what the potential use cases would look like.
Vant: 100%. If you look at what's happened in other industries, what I've been wondering about is not so much how are we going to take today's workflows and change them with generative AI?
(I’m wondering) how are we going to take today's workflows, chop them up into pieces, move them around, do them in different ways, outsource them to different people with generative AI? Because that's what Uber did to taxis.
Uber made it super easy to interface and find a driver. There's no more calling a taxi company and waiting for 45 minutes. They made (taxi) medallions no longer worth hundreds of thousands of dollars because anyone could drive a car.
Uber saw that the power is in having the network and being able to meet – join Kendra, who wants a taxi, with James who's going to drive his car to the airport.
And Airbnb when it came in and said, “You think you have to have hotels that are dedicated just to having hotels and when they're empty, they're empty? No, we think people can have their spare bedrooms and their holiday houses and only put them up available sometimes, and people will be so interested in staying in different venues and destinations, that they'll think it's an adventure to go and find all these funky places to live.”
I'm sure that's what the strategists are looking at is, not how do we drive on the same roads at a faster speed, but are they the same roads?
Do I know what that looks like for the accounting industry? No, not at all.
DigitalFirst: I guess we are really at such an early stage. I was a little disappointed by Intuit Assist when I first saw it, it did feel a bit like a chatbot that does things that you could already do. So it doesn't feel like we've really seen generative AI at work in accounting software. But it raises the question, is this an essential area that we should expect accounting software companies to be exploring at a fundamental level?
Vant: I think it is, I think it is. But yeah, we might all be proved wrong in 18 months. It's just so sexy at the moment, right? Everybody wants to say that they know how to use this for good. It feels almost unbelievable that you wouldn't take a data-rich, small task-rich area like keeping books really well and in some way enhance or transform that with large language models. Now maybe we're right or maybe everybody's collectively deluded. I don't know.
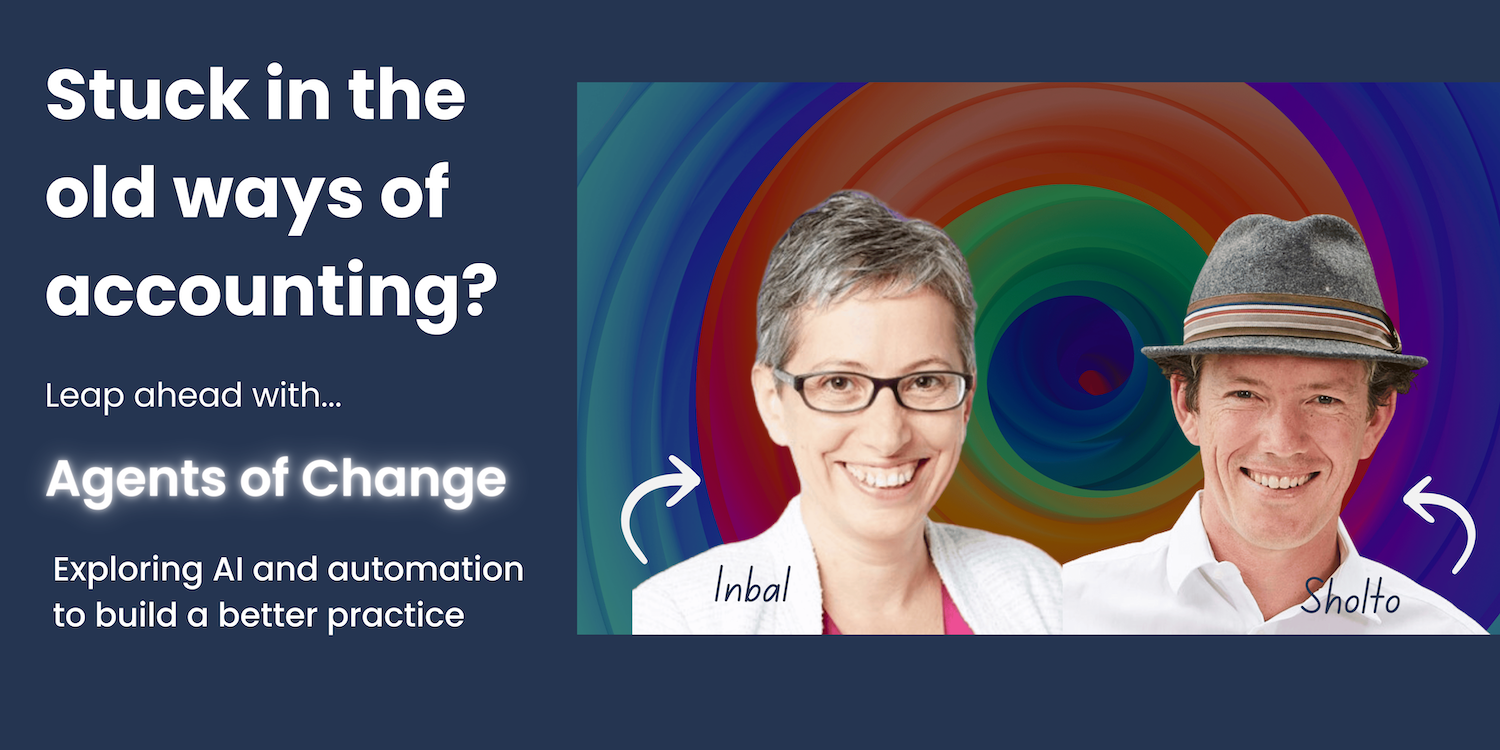